Multimodal Learning for DeepFake Detection
In recent years, the rise of deepfakes—videos that are digitally altered using artificial intelligence (AI) to convincingly depict false events or individuals has sparked significant concerns about the reliability of visual content captured by surveillance systems. This project is driven by the recognition that traditional methods of detecting video manipulation are becoming less effective as AI-driven techniques for creating deepfakes advance. These manipulations can vary from subtle changes to sophisticated fabrications, posing challenges for both human observers and automated systems in distinguishing between authentic and falsified footage. Figure illustrates the facial and foreground/background manipulations.
The consequences of undetected deepfakes in video surveillance are far-reaching, potentially resulting in serious outcomes such as wrongful accusations, compromised security operations, and a decline in public trust in surveillance technologies. Incidents such as the spread of deepfakes of Barack Obama, famous singer Taylor Swift, and many more, caused alarming chaos in social media, reducing the trust in the content. Thus, there is an urgent need to develop advanced detection methods capable of identifying multifaceted falsifications across different dimensions, including visual features, temporal inconsistencies, and contextual clues. This project aims to improve security measures by equipping surveillance systems with the capability to accurately identify and mitigate the impact of deepfake content.
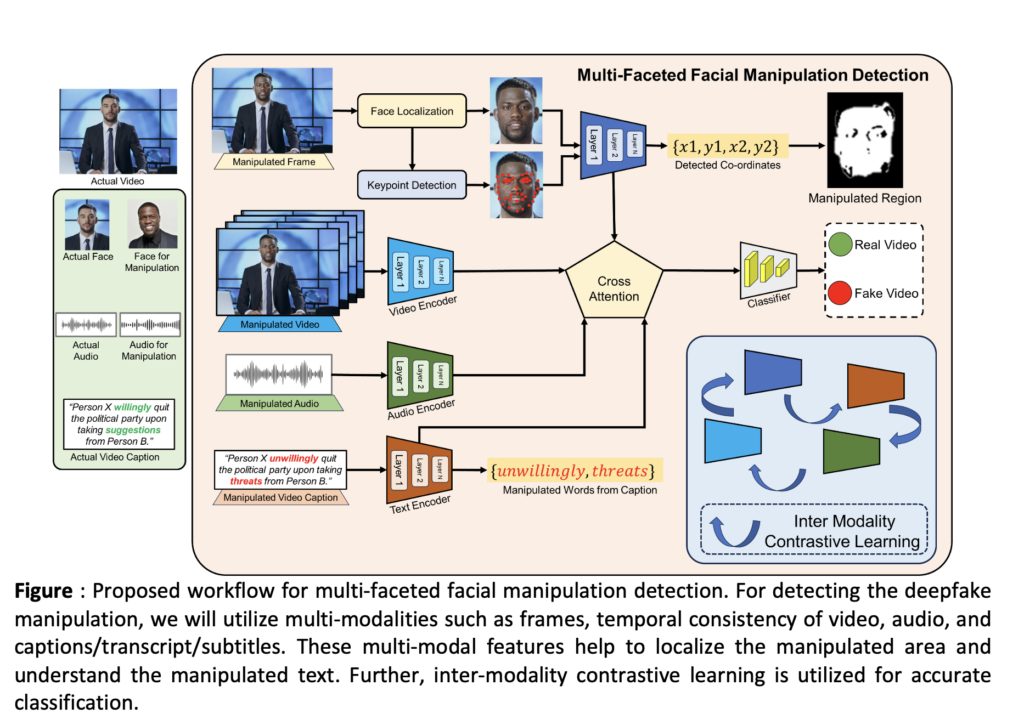